Table of contents
Introduction
Sentiment Analysis is revolutionising the way businesses and organizations understand customer feedback. Leveraging artificial intelligence and machine learning algorithms, we can categorise opinions and feelings expressed in text.
What is Sentiment Analysis?
Sentiment analysis interprets and classifies emotions within text data at its core. It employs a combination of natural language processing (NLP) techniques and machine learning models to sift through sentences. These algorithms identify words and phrases that indicate particular feelings, happiness, sadness, etc. This technology has gained significant importance and popularity over the years due to its applications ranging from customer service to politics.
More than Words
Rather than just identifying keywords in a sentence, sentiment analysis aims to understand the context in which these words appear. For example, the word “good” can appear in a positive context (“The movie is good”) or a negative one (“Good that it’s over”). Algorithms consider these nuances to ensure accurate sentiment derivation. Typically, they categorize the outcome as “Positive,” “Negative,” or “Neutral,” although some models offer a more nuanced classification or a numeric sentiment score.
The foundational work includes text pre-processing and feature extraction. Steps like lowercasing, stemming, and lemmatization prepare the text for analysis during pre-processing. The cleaned text then undergoes transformation into a machine-readable format. Common feature extraction techniques such as Bag-of-Words (BoW) or Term Frequency-Inverse Document Frequency (TF-IDF) convert the text into vectors. Once prepared, various machine learning models like Naive Bayes, Support Vector Machines, or neural networks train on labelled datasets. They learn the correlation between text features and their corresponding sentiments.
Handling the subtleties in human expression poses one of the biggest challenges in sentiment analysis. Misunderstanding sarcasm as genuine praise or criticism is easy. Additionally, idiomatic phrases and contextual usage make the landscape more complex. Modifiers can dilute or amplify sentiment. For instance, “The meal was okay” and “The meal was incredibly okay” carry different sentiment weights, even though “okay” defines both.
Different Aspects of Sentiment Analysis
Different types of sentiment analysis address different needs:
- Fine-Grained Analysis: This approach categorizes sentiment into more specific classes, such as “Very Negative,” “Negative,” “Neutral,” “Positive,” and “Very Positive.”
- Aspect-Based Analysis: In this approach, the algorithm identifies various aspects or features mentioned and categorizes sentiment for each. For example, in the review “The phone has an excellent camera but a poor battery,” the sentiment towards the camera is positive, and towards the battery, it’s negative. This is a more advanced form of comment analysis.
- Emotion Analysis: This form identifies specific emotions like happiness, anger, or sadness. It proves especially useful in contexts where understanding emotional tone is crucial.
- Intent Analysis: Used mainly in customer service scenarios, this type identifies customer intentions such as buying, leaving, or seeking support.
What does the Future Hold?
The adaptation of AI to analyse voice tonality and facial expressions is ongoing. This holistic approach offers a more in-depth understanding of sentiment but comes with its own set of challenges, such as accurate voice and facial recognition.
In academia, sentiment analysis remains a hot research area. Researchers are addressing challenges like creating high-quality labelled datasets for training, dealing with imbalanced classes of sentiment, and understanding increasingly complex human sentiments and idioms.
In summary, sentiment analysis tools combine linguistics and computer science to decode subjective information. As computational models become more sophisticated and our understanding of human emotion and language deepens, the scope and significance of sentiment analysis will continue to grow. It will offer nuanced and context-rich insights into our textual communications.
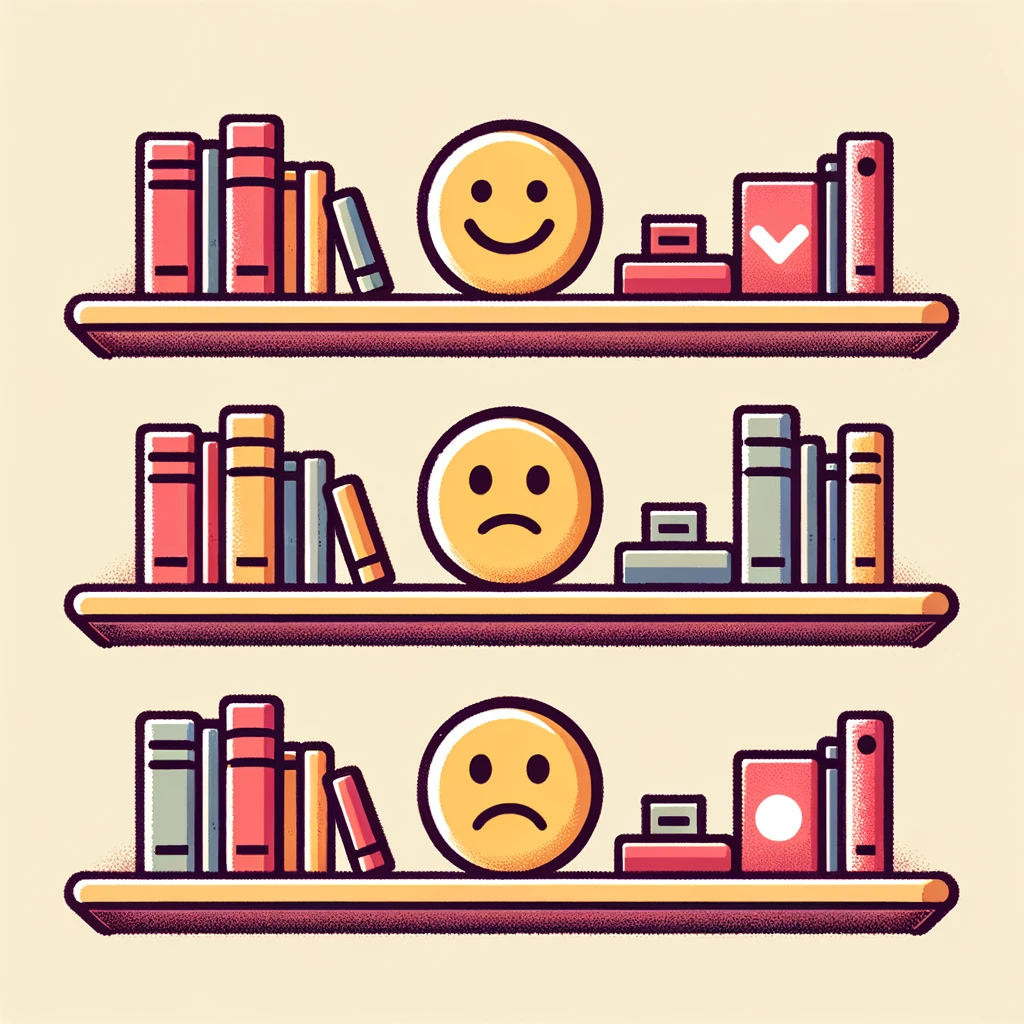
Why is Sentiment Analysis Important?
- Customer Feedback: Understand how your customers feel about your products or services with easy customer sentiment analysis. Andach Insights can link into your reviews on Amazon, eBay, Shopify and more platforms, providing you with clear, actionable insights to drive revenue growth.
- Staff Retention: Any HR manager knows that proper use of exit interviews is critical to reducing staff turnover, and associated hiring costs. By using Andach Insights to analyse exit interviews, you can identify trends quickly and simply. That frees you up to get on with the job of making your company an inviting and inclusive place to work.
- Brand Management: By monitoring comments on social media feeds you can identify extreme negative or positive sentiment and capitalise on this immediately while it’s hot. Today’s news is famously tomorrow’s fish and chip paper, but social media moves even quicker than that. Even a few hours can make the difference between capitalising on a viral tweet and missing the mark.